Formatting and editing by Madeline Rizzo, J2 Interactive
Part One | Part Two |
---|
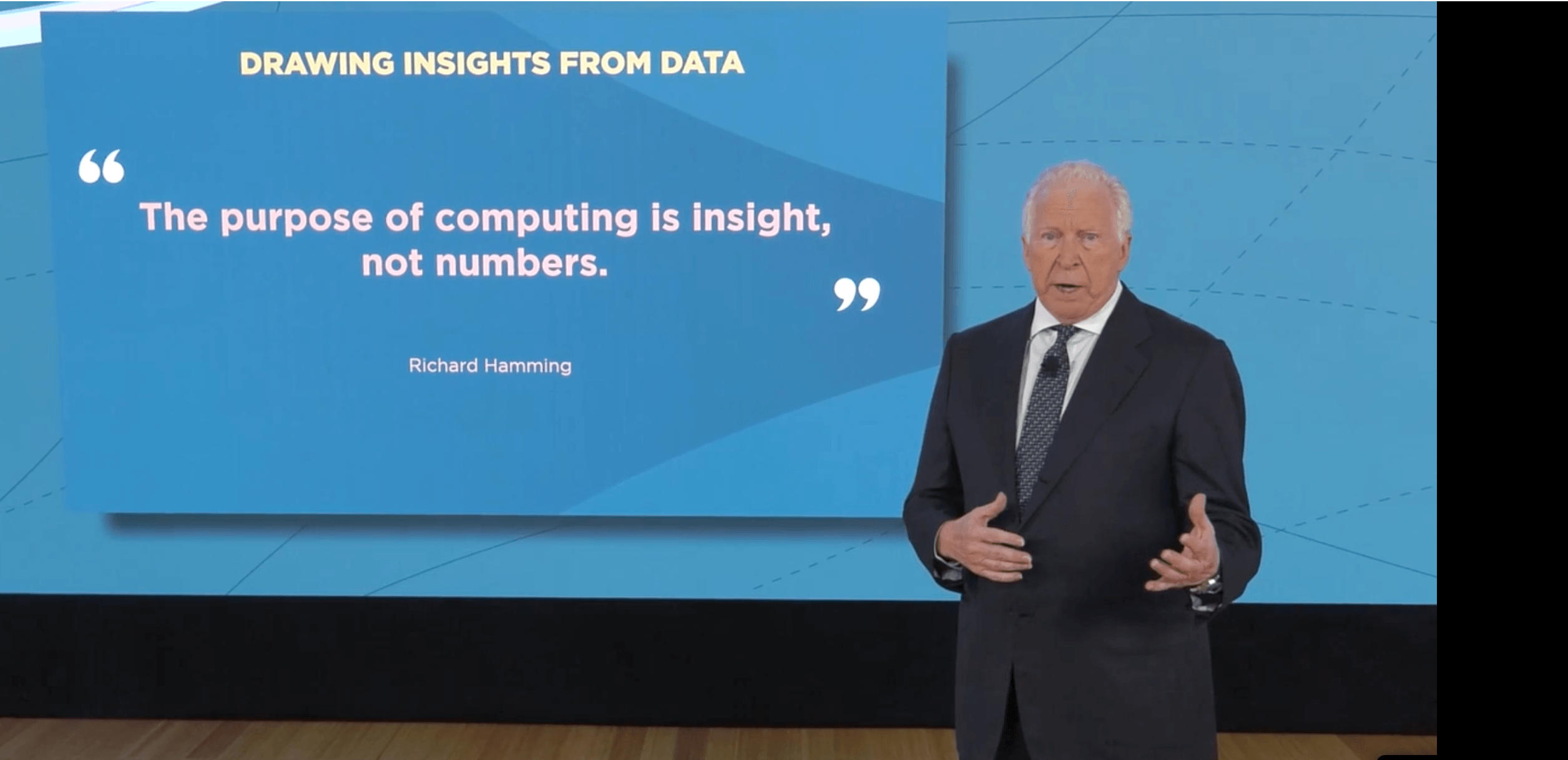
2021 marks the second year of InterSystems’ Global Summit going virtual! We attended and are pleased to provide highlights from some of our favorite sessions. Continued from part one!
What’s on the Horizon for Payer Interoperability?
Summary by: Jen Carter, J2 Interactive
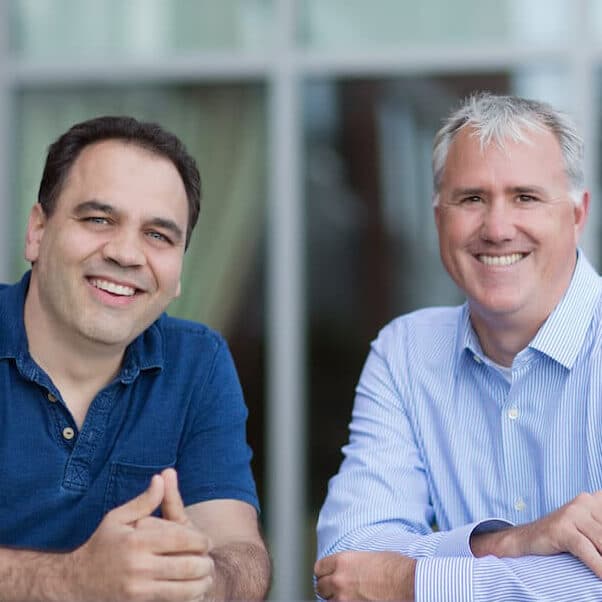
- Date: October 27, 2021
- Presenters:
- Lou LaRocca, President and CEO, J2 Interactive
- Mickey Yalon, Chief Technology Officer, J2 Interactive
- Overview: By taking advantage of the integration and API management capabilities of InterSystems IRIS for Health and the InterSystems IRIS FHIR Repository, SCAN Health Plan became one of the earliest payers to meet the requirements of the CMS 9115 Final Rule. In this presentation, J2 Interactive discusses how they and payers like SCAN are preparing for the next round of CMS interoperability requirements and use cases.
InterSystems implementation partner J2 Interactive has seen increased interest in interoperability among payers over recent years. Clients like SCAN Health Plan find themselves relying on the InterSystems platform not only to meet regulatory requirements but strategic goals as well.
Access to patient data has been a historic challenge in healthcare. CMS’s patient access final rule, known as CMS 9115, aims to improve patient care across the country by providing access to patient data and empowering patients to choose among multiple health plans. The rule also specifies that interoperability between payers should be a priority, thus enabling consumers more freedom to access the care that’s best for them.
SCAN Health Plan, located in Southern California, is one of the country’s largest not-for-profit Medicare Advantage plans with a particular focus on care for seniors. SCAN was one of the first health plans to meet the CMS 9115 Patient Access and Provider Directory rules by leveraging the IRIS for Health Data Platform.
While committed to meeting the regulations, SCAN wanted to take advantage of the full potential of the IRIS Data Platform. They saw this implementation as a way to leverage data across the entire enterprise to enable future growth and strategic initiatives. In order to achieve this, it was important to SCAN that their implementation partner was fully committed to building internal expertise on the platform.
SCAN chose IRIS for Health because it was a robust, performant, long-term platform for future use cases, not simply for meeting the requirements of 9115. Support for FHIR r4 was particularly appealing to the organization.
Support for payer to payer data exchange capability is up next for CMS 9115. The requirement from CMS is not as specific as the previous requirements, leaving health plans to decide how to exchange data, which creates a challenge since payers are left to select their own standards. In response to that feedback from payers, CMS has pushed the rule back, and we expect to see a more detailed specification, probably one requiring support for FHIR r4, but that’s still to be finalized.
Health Connect: New & Next
Summary by: Stuart Byrne, J2 Interactive
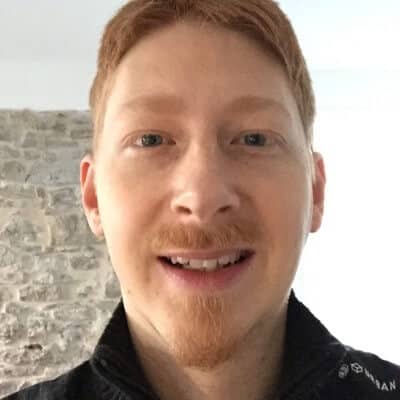
- Date: October 27, 2021
- Presenter: Stefan Wittmann, Product Manager, InterSystems
- Overview: Stefan Wittmann, Product Manager at InterSystems, talks to the audience about the upcoming enhancements for Health Connect.
Stefan Wittann, Product Manager at InterSystems, talked to the audience about the upcoming enhancements for Health Connect.
These enhancements include:
- FHIR capability development
- Continuation of support for FHIR R4
- Future support for FHIR R5
- Development of FHIR accelerator service to aid getting data into and out of FHIR resources
- Release of FHIR Resource repository for the storage and documentation
- Improved API management allowing fine control of FHIR APIs
- New support for IHE profiles:
- RMD profile support – metadata removal from doc registry and doc repository
- RMU profile support – document sharing metadata modification
- Property forEach handling in business rules
- Future release of New look Angular Business rule editor
- Automation tools for configuration:
- HL7 Productivity toolkit
- HL7 DTA generator
- HealthShare Message Transformation Service
IntegratedML Update from the Field
Summary by: Mark O’Reilly, J2 Interactive
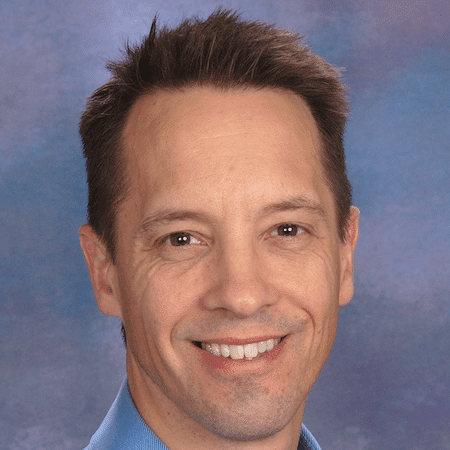
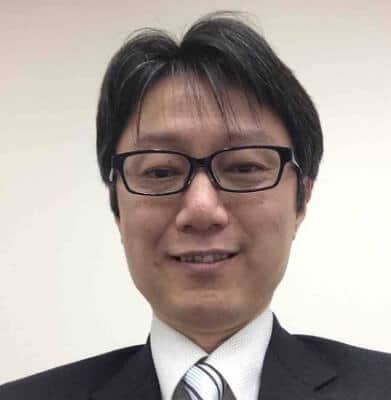
- Date: October 27, 2021
- Presenters:
- Thomas Dyar, Product Specialist, InterSystems
- Minoru Horita, Manager Sales Engineering, Japan, PALTAC
- Overview: Thomas Dyar, Product Specialist at InterSystems, and Minoru Horita, Manager of Sales Engineering at PALTAC speak about Integrated Machine Learning, which is currently available on the InterSystems IRIS platform. The two illustrate how Integrated Machine Learning can be used in InterSystems IRIS using real-world examples and discuss future enhancements for InterSystems IntegratedML.
In this presentation, InterSystems Product Specialist Thomas Dyar outlined the use of Automated Machine Learning (AML) and how it is built into InterSystems IRIS. Dyar offered a brief look at how models were created and the engines that could be used in conjunction with IRIS to make predictions.
If you are not familiar with Machine Learning or Automated Machine Learning, here are some basics:
Automated Machine Learning Basics
As IBM states, “Machine learning is a branch of artificial intelligence (AI) and computer science which focuses on the use of data and algorithms to imitate the way that humans learn, gradually improving its accuracy.” Automated machine learning uses software rather than user-specific developed AI systems to make predictions based on data.
IntegratedML
IntegratedML is a “SQL facility” and uses simple SQL statements (on most data platforms) to create/train data models and make predictions that can be used in your SQL. Simple SQL statements then are the building blocks for machine learning software, which uses the data to build the AI. There are some default tools used in this process; however, there are also other pluggable ML engines available. We will come back to the ML engines later.
As an example of IntegratedML, the presenters discussed having an e-commerce company. With an e-commerce company, there are many random delays, and you’re not sure exactly why these delays may be happening. Related data can thus be collected within IntegratedML and help predict delays.
First, the CREATE MODEL command sets up the machine learning model metadata. In the image above DelayModel is the name of the model. Delay is the field we want to make a prediction about, and OrderView is the dataset used to infer the input and target fields.
The TRAIN MODEL command specifies the data to be used for training and executes the AutoML engine, which takes a set of relational data as input.
You can query the machine learning validation metrics table once the model is trained to see metrics on how accurate the trained model is.
InterSystems has multiple types of engines that do machine learning.
- The default is AutoML. It is useful for people who just want to get started right away, in addition to being good for prototyping.
- H2O can be used, which is also free, like AutoML. It has more configurable options, but a downside is that it ignores Text columns.
- DataRobot is not free but is a class-leading AutoML engine. You can log in to the DataRobot platform itself to analyze the model’s performance and use a host of features.
Real-World Examples
Healthcare
In Houston, InterSystems is using data based on information from the Greater Houston Healthconnect instance, a Health Information Exchange (HIE) based in the area, to predict the likelihood of a person either becoming diabetic or, based on data, how their issue with diabetes will be six months in the future.
This project is still ongoing, but it hopes to demonstrate the benefit of using automated machine learning for healthcare predictions that would be beneficial to both doctors and patients.
Financial Services
In the financial services field, InterSystems worked on predicting whether a customer would make a large withdrawal (over 50% of current balance) based on previous transactions.
InterSystems initially trained the AI with 2019 data and then evaluated it every month in 2020 to see differences between the monthly model predictions vs. what actually happened. Each month, they then retrained the model based on new data gathered.
The model was very successful apart from evaluating older customers, where there was a lack of data.
PALTAC
PALTAC distributes cosmetics, daily necessities, and medicine to drug stores, convenience stores, supermarkets, and home centers. They currently use InterSystems IRIS with Integrated ML using DataRobot to predict demand for products. The company uses AutoML because of growing competition and the need to save costs by reducing food/water loss.
The company is currently in a proof of concept to improve demand forecasting by 10%. They are using data from 30 out of 1000 stores for their model. DataRobot is being used as their AutoML software.
Already, they have improved predictions by 5%, and there is further work to be done to improve the models. These improvements include using a new feature in InterSystems IRIS, integrated Python, to integrate with the DataRobot API and improve the models even further.
Future Directions
InterSystems plans to do the following in the future:
- Support time series in modeling, which will help with particular forecasting models. They will support DataRobots AutoTS in addition.
- Make AutoML more extensible, for example, allowing developers or data scientists to modify bits of the ML process by Python or another mechanism.
- Create packages to inspect the model and determine what variables were important for a particular prediction with the idea to bring back a table of the contributions to show why a prediction was made.
- Manage ongoing training commands.
- Import and export models.
- Conduct efficiency improvements for larger datasets.
Try Extended Decision Support: Real-Time Rule-Based Alerts for Clinicians – Live Session
Summary by: Luis Sayago, J2 Interactive
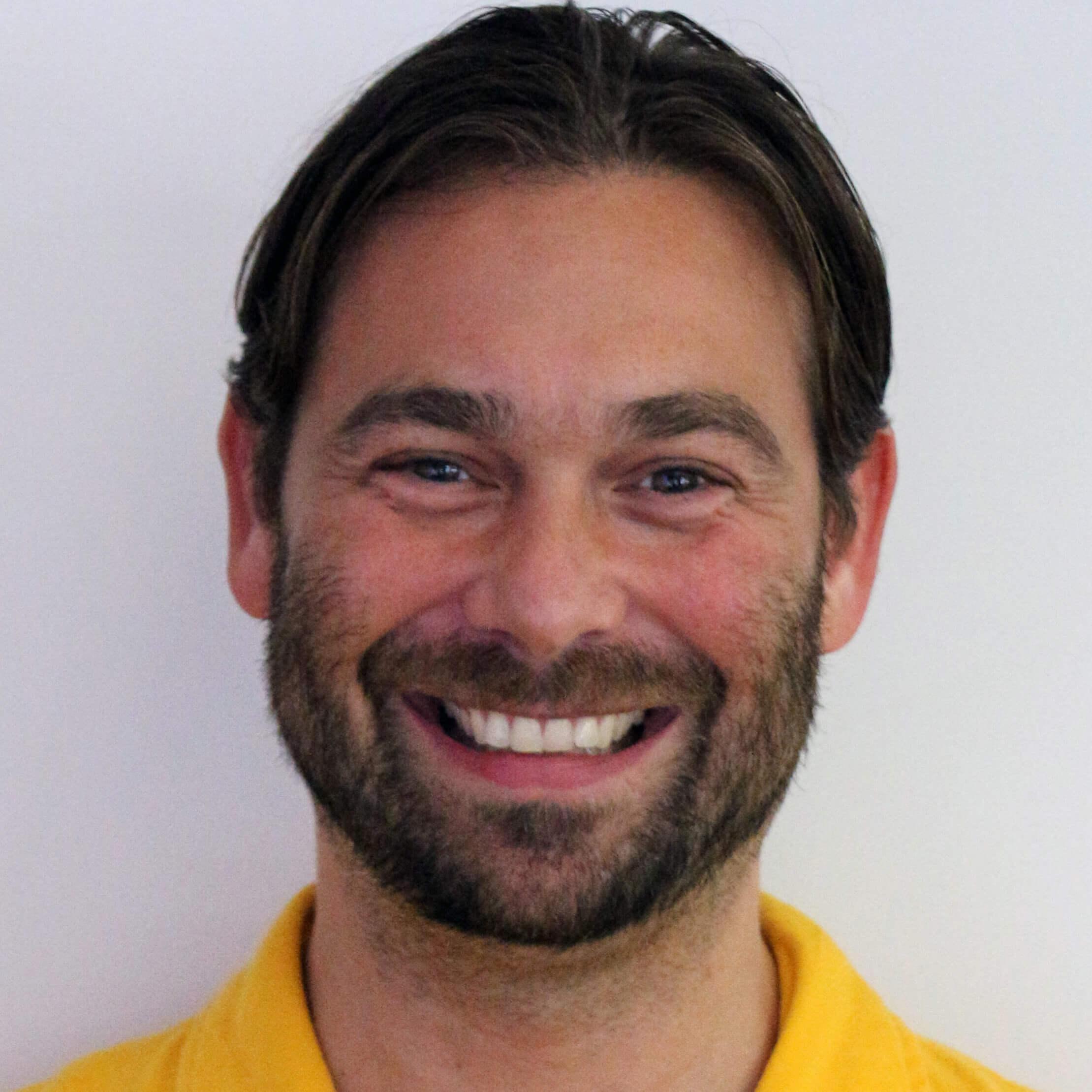
- Date: October 28, 2021
- Presenter: Frank Pandolfe, Clinical Product Specialist, InterSystems
- Overview: Frank Pandolfe, Clinical Product Specialist at InterSystems, leads a lab where participants create a CMS rule-based alert in the Rule Editor and a clinician-facing card. Participants then test their alert by triggering the rule, receiving the alert as a clinician, and choosing to accept or reject the suggestion on the card.
This lab focused on upcoming HealthShare Extended Decision Support (EDS) functionality, a new product by InterSystems, currently in the pre-release Beta. HealthShare EDS uses data from patient records to generate real-time, actionable notifications for clinicians and supporting applications.
First, Pandolfe covered the basic EDS architecture, which is a reference implementation of the CDS Hooks specification. Applications that support CDS Hooks, within and outside of HealthShare, can take advantage of EDS logic without any need for clinicians to leave their workflow.
Next, the lab moved on to the hands-on portion, starting with the setup of the learning environment. After that, we jumped into tasks including “Create a CDS Hooks Card,” “Create a New Rule,” and “Assume the Clinician Role.”
As the analyst role, building the card look and behavior as well as rules was straightforward using the “no-code” HealthShare web interface. The rule editor allowed for using data in the patient’s record or building a FHIR Query.
In closing, Frank Pandolfe answered some questions about the lab and the product. I asked if he could expand on how the EDS cards would be placed in the clinician’s workflow, and he answered by saying that any client application that supports CDS Hooks can display cards generated by EDS.
Existing customers can request the Beta version as POC for their site, but they will need to have a FHIR Gateway available. Release tentative in 6 months in 2022.1.
Embedded Python for ObjectScript Developers
Summary by: Emily Lupton Metrish, J2 Interactive
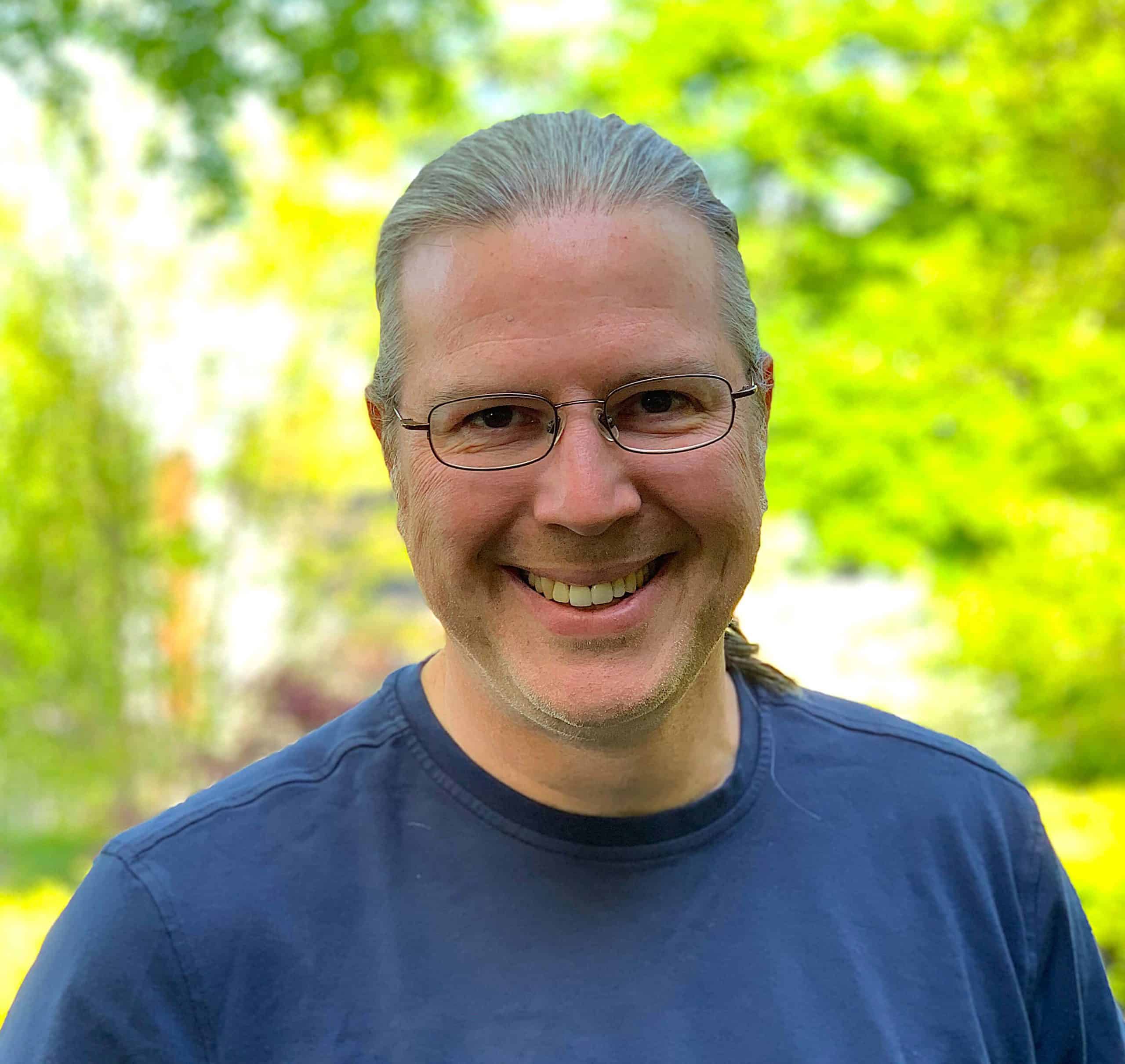
- Date: October 27, 2021
- Presenter: Bob Kuszewski, Project Manager, Developer Experience
- Overview: As a follow-up to the Introduction to Python presentation, this one primarily gives examples of Python embedded in Caché ObjectScript and offers a few demonstrations of specific APIs.
IRIS uses what Bob Kuszewski, Project Manager at InterSystems, refers to as a “language bridge” between Python and ObjectScript compilers. This bridge allows Python to run embedded within ObjectScript code (check out the $system.Python library). Essentially, you can create a program in Python and then call it using ObjectScript code. However, you need to be careful, as there are differences in what COS and Python accept in terms of variable/class names. Most variable types are converted automatically, but some things (like lists) need to be explicitly converted when passing in.
Kuszewski instructed people to use $system.Python.Shell() to start a built-in interactive Python shell. If you type “import iris,” you can then load and run classes. The example he provided was a COS class for normalizing an address with the notation [language = python] in the method header, and the actual class is in Python. There are also similar issues with characters allowed in COS but not in Python, like the %.
Exploring Machine Learning with R Language Gateway
Summary by: Royal Sefton, J2 Interactive
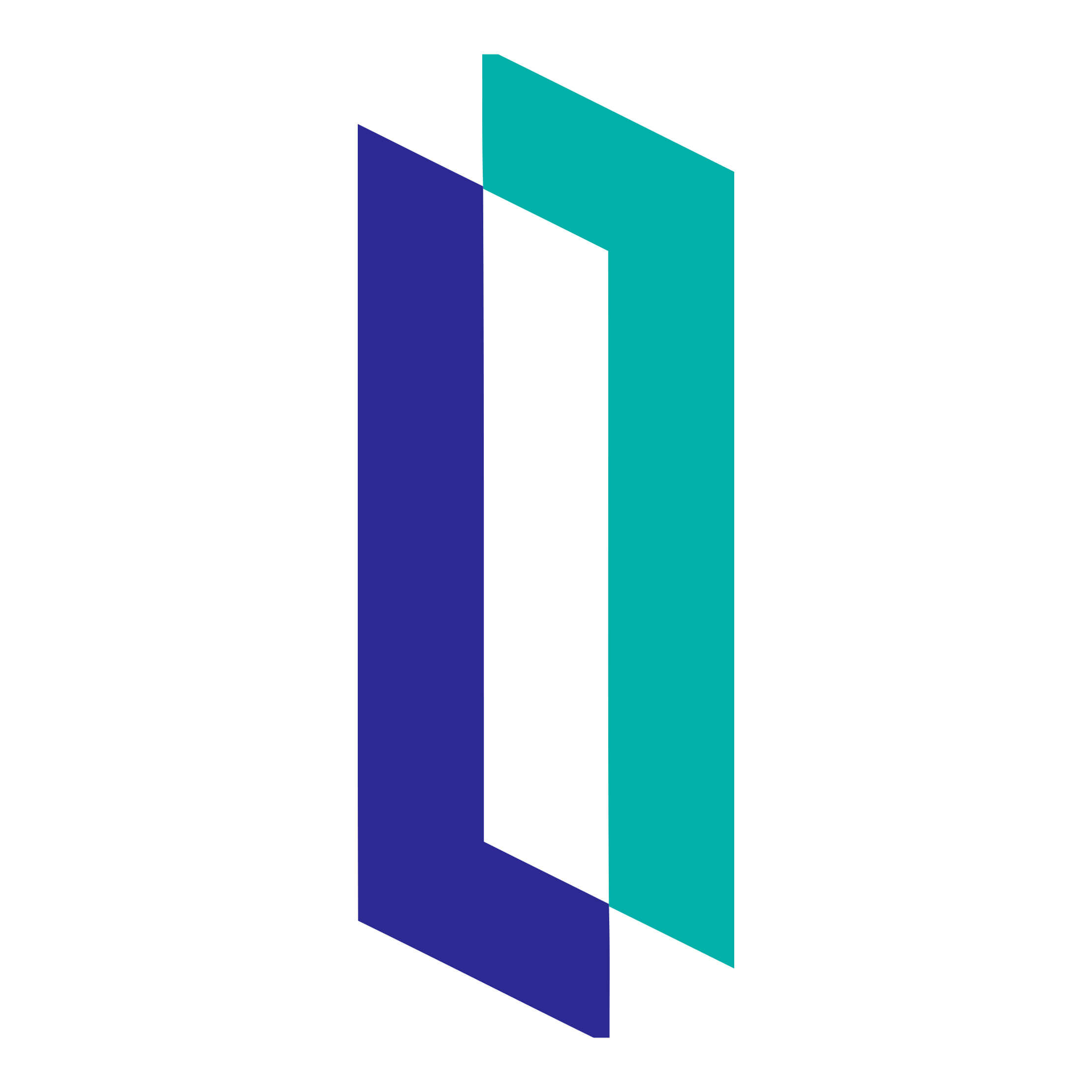
- Date: October 27, 2021
- Presenter: Shiao-Bin Soong, Systems Developer, InterSystems
- Overview: InterSystems now provides an R Language Gateway with IRIS for Health 2021.1, which allows developers to connect IRIS with R and take advantage of its unique capabilities in data analytics, statistics, and machine learning.
With IRIS for Health 2021.1, InterSystems has added an R Language Gateway. R is a language best suited to data analytics, statistics, and machine learning tasks and is widely used by data scientists and informaticists. The inclusion of this R Language Gateway gives IRIS developers the ability to communicate with an R Server by way of the free and open RServe project (using the REngine class), enabling inline evaluation of R code in ObjectScript.
InterSystems demonstrated this inline evaluation, along with the communication of values between IRIS and RServe in the construction of a confusion matrix and resultant machine learning model, with a modest dataset concerning a sample set of flowers (irises, specifically) and their recorded measurements. The demonstration concludes with the creation of a data frame from the dataset in IRIS SQL, transmission of the data frame to R, the creation of a data model, and the evaluation and testing of said model. In essence, this is a demonstration of how one could extract data from IRIS and feed it directly into R for analytics and machine learning, thereby harnessing R’s strengths from within IRIS Studio.
Adopting the R Language Gateway into one’s data science toolkit will enable data scientists and informaticists to quickly leverage the power of R in creating data models (both quick and presentable) and calculating statistics from rich clinical data from within IRIS Studio, bypassing any intermediate extract-transform-load (ETL) steps in the data analytics workflow. R also has more than 15,000 packages available via the CRAN (Comprehensive R Archive Network, analogous to Perl’s CPAN), making it incredibly extensible and nearly limitless in its potential. With the R Language Gateway, these packages essentially become available to IRIS developers, yielding a tremendous potential for all manner of data munging and analysis.
Prior Authorization: A Shared Provider/Payer Project
Summary by: Stacy Welton, J2 Interactive
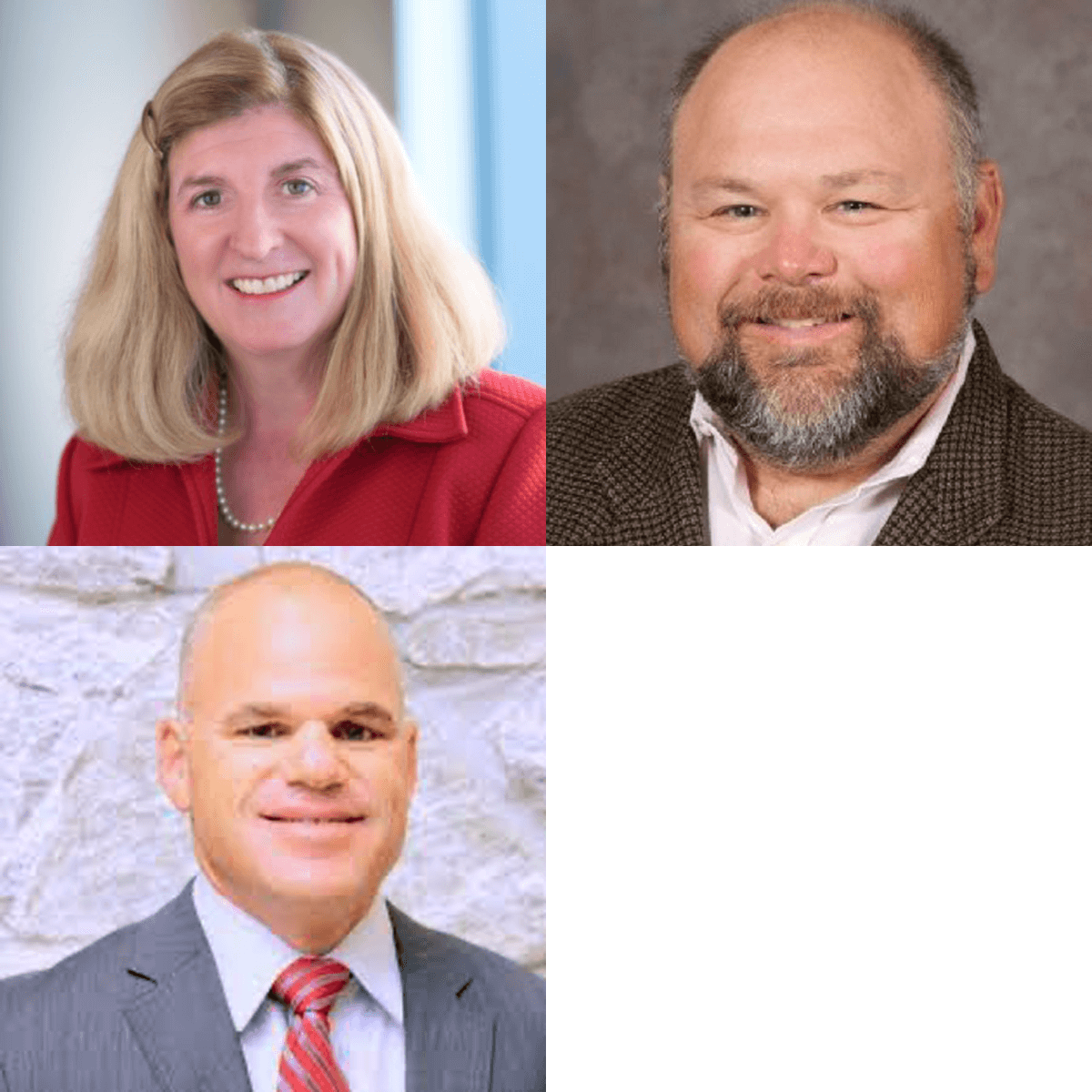
- Date: October 27, 2021
- Presenters:
- Lynda Rowe, Senior Advisor – Value Based Markets, InterSystems
- Michael Marchant, Director, Health Information Exchange and System Integration, UC Davis Health
- Jonathan Copley, Sr Director, Interoperability Strategy & Innovation, Centene Corporation
- Overview: InterSystems, UC Davis, and Centene tackle the “thorny” challenges of prior authorizations as part of the Da Vinci Project. “Da Vinci is a private sector initiative that addresses the needs of the Value Based Care Community by leveraging the HL7 FHIR platform.”
In this presentation, presenters from InterSystems, UC Davis, an academic hospital system, and Centene, a managed care organization, discussed how prior authorizations and an endeavor by the Da Vinci Project improved patient/member experience, reduced costs, and streamlined provider/payor data exchange.
Prior authorization is “a management process used by insurance companies to determine to what extent prescribed products or services, such as medications or procedures, are covered prior to the patient receiving them.” Billions yearly in costs associated with latency (lack of real-time flow), manual work, redundant efforts, and overhead between providers and payers are all “burdens” of prior authorization.
Enter a new collaboration, Prior Authorization Support (PAS), leveraging InterSystems’ HealthShare for real-time connectivity, brokering transactions, and applying rules & security to the transactions that are shared with Centene to process the prior authorization data from UC Davis’ EMR Epic. This process is accomplished first with InterSystems “Clinical Decision Support Hooks” into the Epic chart. That data is transmitted to Centene as a prior authorization request using CQL – Clinical Quality Language, where special “repositories” of data are run against the hooked transactions. Centene then responds back with a yes, no, or a request for more information.
In 2020, CMS released a proposed rule that prior authorization provider/payer interoperability will be required in 2023. Our friends in The Da Vinci Project are already ahead of the game.
Parsing Images and Charting Data with Embedded Python – Live Session
Summary by: Luis Sayago, J2 Interactive
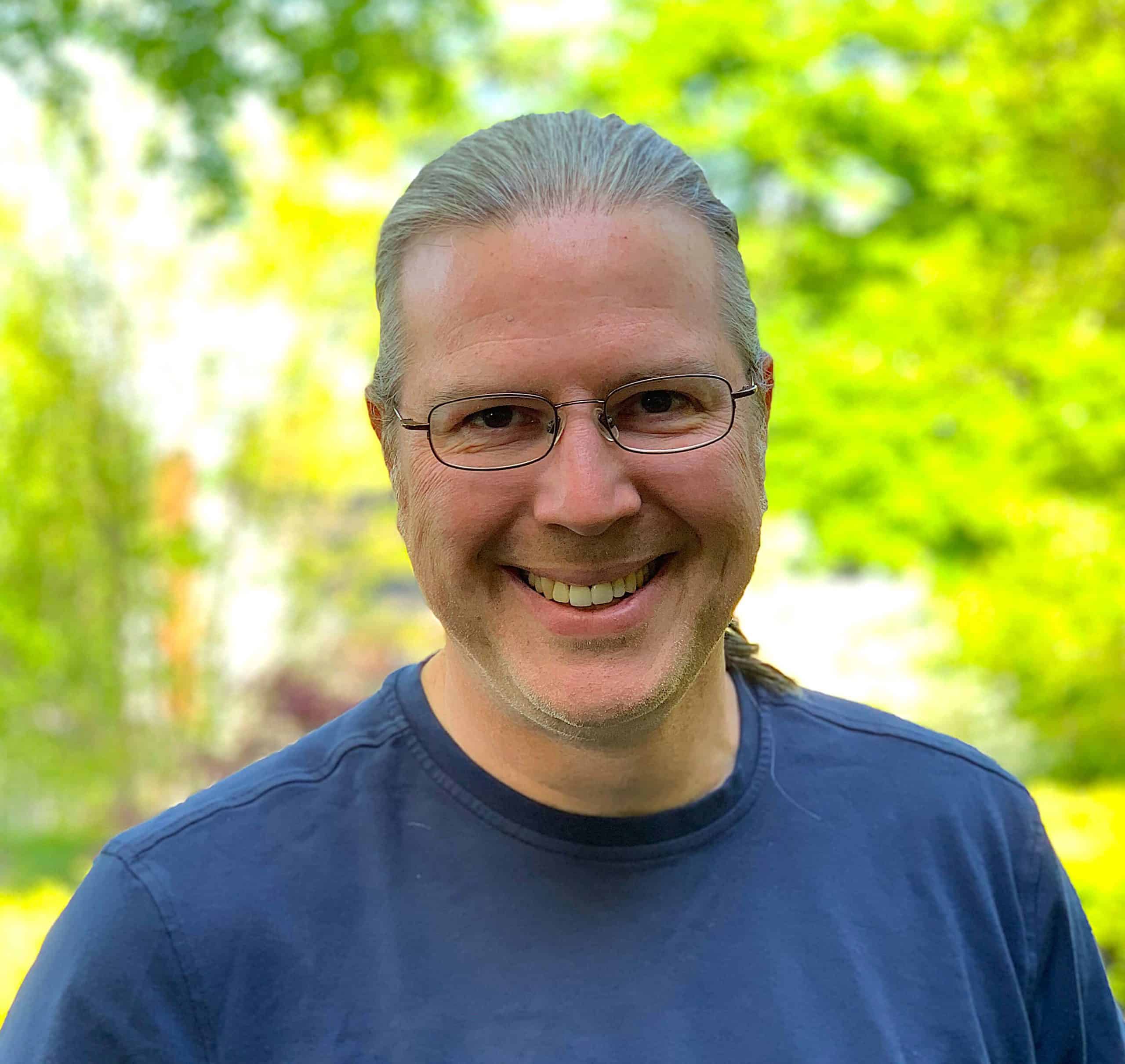
- Date: October 28, 2021
- Presenter: Bob Kuszewski, Product Manager, at InterSystems
- Overview: Bob Kuszewski, Product Manager at InterSystems, leads viewers through a lab in developing an expense-tracking application that uses open-source Python libraries to parse images of receipts, store them in a central database, and graph total weekly expenses.
This lab focused on demonstrating the upcoming Embedded Python functionality, a new feature of the InterSystems IRIS data platform. Bob Kuszewski provided some technical details and introduced us to the “Expense Reimbursement” application that we would be building.
Some tasks the application performed included:
- Parsing receipt images (Using Native Python CLI)
- Automatically running currency conversion (Using Python Library CurrencyConverter embedded in ObjectScript)
- Storing receipt data in the InterSystems IRIS database (Using IRIS Python library to persist objects)
- Exposes a REST API endpoint that returns a bar chart of weekly expenses (Using Python, and Python libraries like matplotlib, in an ObjectScript Class Method)
I asked Bob in the Q&A if we would be able to run Jupyter Notebooks using Embedded Python, and he replied that we would. They are finalizing some documentation that will be available with the official release.
Embedded Python will be available in the next IRIS release 2021.2, including Community Edition. For HealthShare, it will be available in 2022.1, which is the next release to include the latest IRS long-term support version.
Introduction to FHIR SQL Builder
Summary by: Dawn Tracey, J2 Interactive
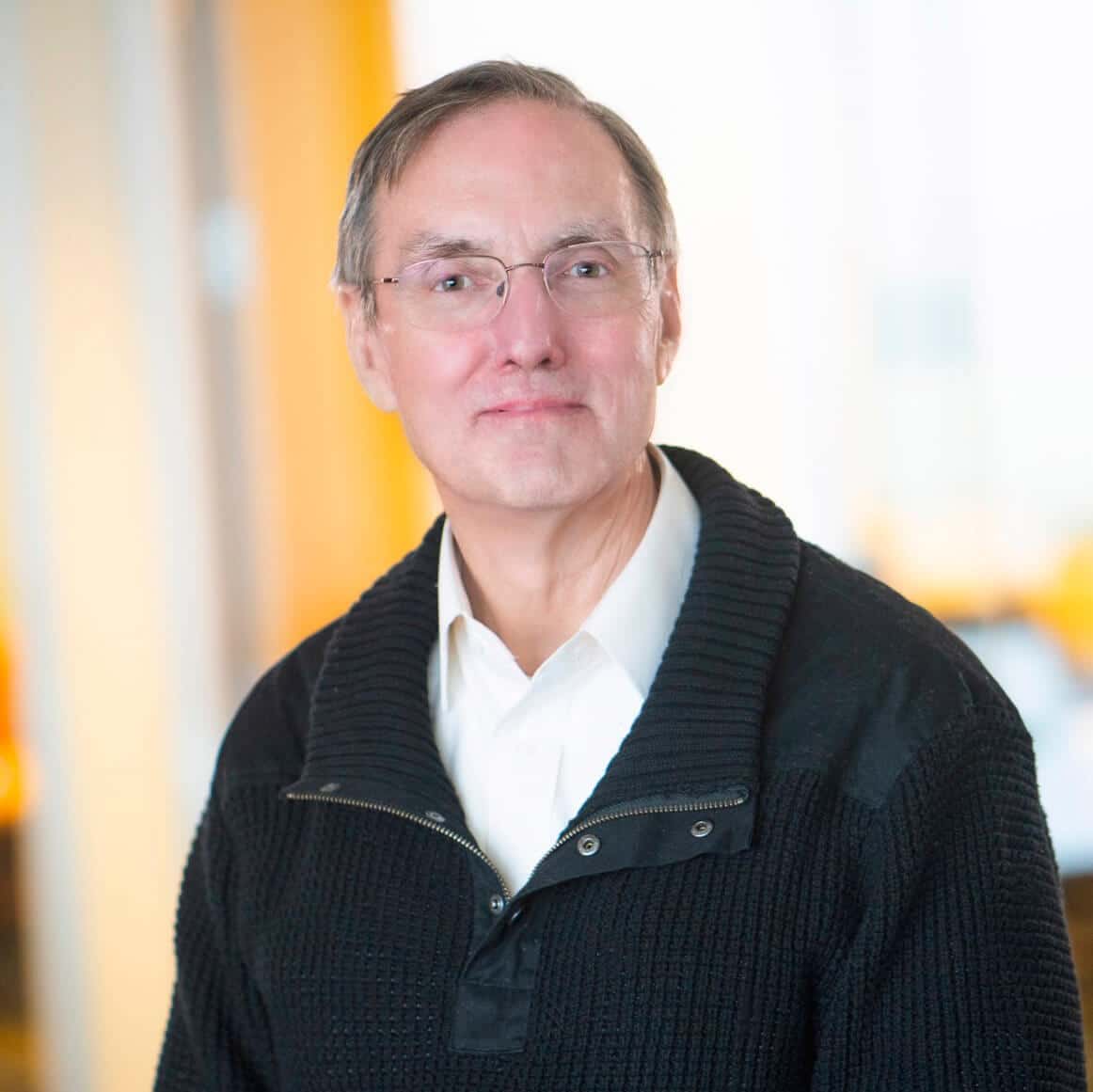
- Date: October 27, 2021
- Presenter: Patrick Jamieson, M.D. Product Manager, IRIS for Health
- Overview: Dr. Jamieson, a Product Manager for IRIS for Health at InterSystems, presented a (not yet released) product that will enable users to easily access data from their FHIR repository and analyze it using familiar analytic tools.
As FHIR becomes more widely used in healthcare, accessing and analyzing FHIR data is going to become ever more important. Current processes to do this require complex technical skills not widely available. InterSystems has developed a product that will allow users to easily access data from their FHIR repositories and analyze it using familiar analytic tools.
The FHIR SQL Builder will take the non-relational FHIR resource data and present it to the user allowing them to create a custom relational SQL schema.
Step 1. Analyze data in FHIR repository
Step 2. Create transform specification
Step 3. Transfer data to SQL Table
Step 4. Access data
As noted earlier, this product is not yet available but InterSystems is implementing an early access program. If interested contact Patrick.Jamieson@intersystems.com.
Part One | Part Two |
---|
Thank you for joining us for this year’s InterSystems Virtual Summit recap!
J2 Interactive is an award-winning software development and IT consulting firm specializing in customized solutions for hospitals, labs, research institutions, and health information exchanges.
Our approach to design and development is rooted in a fundamental belief that systems succeed or fail based on how well they serve the people who depend upon them. Drop us a line to learn more.